As stated in
Part 1 of this article, my first step in making an informed decision on the subject was to uncover as many facts as possible. Although one would think this would be easy, reality proved otherwise, as most of the Google results I looked at were clearly biased, taking positions on either side of the debate, then presenting facts to support that position. Such
confirmation bias is not surprising; it is human nature. That's how our
brains evolved, and a hallmark of solid, critical thought is the ability to minimize these biases. The preexisting beliefs I outlined in Part 1 shouldn't drive what evidence I choose to look at, or how I interpret that evidence.
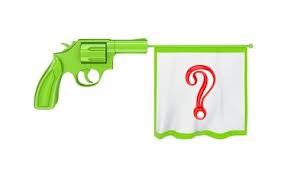
One particularly useful source of information is the article
Gun nation: Inside America's gun-carry culture published March, 2012 in the Christian Science Monitor, a paper known for its original reporting and fairly well-balanced perspective. From this article we learn several facts, including: 1) Gun-rights and gun ownership have expanded significantly over the past 10 to 20 years. For example, the number of concealed-weapon license holders in the US has gone from a few hundred thousand ten years ago to more than six million today; 2) Violent crime and intentional homicide have declined precipitously over the same time period. We are actually living in the most non-violent period in US history; 3) Because of this, not a single scholar in the field will claim that legalizing concealed weapons causes a major increase in crime; and 4) Recent Supreme Court decisions and the Obama administration's own policies have actually buttressed the right of Americans to own weapons; not hindered them. I found all of these facts non-intuitive, and they are exactly the opposite of what most people believe.
Another relatively objective source of information is an
interview with Harvard psychology professor and author Steven Pinker, whose book
The Better Angels of Our Nature: Why Violence Has Declined also turns conventional wisdom on its head. He points out that the rate of violent crime and intentional homicide in the US are about
half of what they were twenty years ago despite increasing gun ownership and increased popularity of violent video games. He tells us that the rate of mass murder incidents has not increased since the 1920s, contrary to popular belief which is driven in part by improvements in communication and instantaneous reporting of news from any part of the world. In short, more people than ever go about their normal lives never being affected by a crime or violence. Finally, he cautions that our discussion about violence and murder should be focused on the 16,000 people who are murdered per year (45 per day) in the US that we never hear about, as opposed to the relatively few who are murdered in rampage killings like Newtown, as such incidents are still so rare that they are nearly impossible to predict and determine the cause.
The final part of my discovery process was compiling data and doing my own analysis. The sources above focus on the US, but it is a big world, and it is meaningful to look at homicide rates by country across a number of independent variables. I chose four variables which the literature and public commonly talk about as potential root causes of violent crime and homicide. They are:
1. Rate of gun ownership (G). Yes, people in other countries do own guns, and murders do occur.
2. Annual video game sales per person (V).
3. Income inequality (I) defined as the average annual income for the wealthiest 20% divided by the average annual income of the poorest 20%, or R/P 20%.
4. Percentage of the population that are males between the ages of 18 and 24 (M), as this group is more likely to commit murder than any other.
I considered including the use of assault-style weapons, but data was not readily available and even in the US, I learned that these weapons are used in a small percentage of all murders committed (2% to 7%, depending on the source).
Comparison nations are those with standards of living and lifestyles most similar to the United States; particularly Canada, most European nations, Australia, New Zealand, Japan, and South Korea. Data, shown in Table 1, comes from a variety of sources (noted below). Although this data is not rigorously vetted and may not be authoritative, I am assuming it is reasonably accurate for my purposes.
The data was analyzed using multivariate regression after normalizing each variable from 0 to 1 so that resultant coefficients could be directly compared. Prior to analysis, the distribution of each variable was qualitatively looked at, and all appeared reasonably normal except for the two highlighted values (gun ownership in the US, and video game sales in South Korea). Because of this, the analysis was performed both with and without the US and South Korea. Results without the US and South Korea are shown in Table 2. Coefficients for G and V are very small and have insignificant t-Stats, meaning the rate of gun ownership or video game sales has no measurable relationship to the homicide rate. Coefficients for I and M are larger, and they have marginal t-Stats, meaning income inequality and percent of the population that is young and male may explain some of the murder rate, but not much of it. The adjusted R-squared value of 0.22 is consistent with this conclusion, indicating that the 4 variables explain just 22% of the overall homicide rate across all countries. Inversely, almost 80% of the homicide is rate explained by something other than these 4 variables (or perhaps some combinations of these).
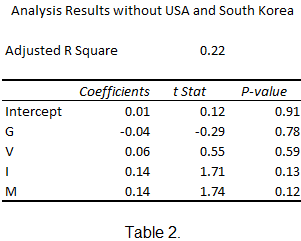
When we add the US and South Korea to the analysis (Table 3), the results become more complicated. Note that the adjusted R-squared value increases to 0.54, meaning the 4 variables now explain up to 54% of the overall homicide rate across countries. Coefficients for I and M also increase considerably, while t-Stats remain marginal. This means that the effect of income inequality and a young, male population may be larger than we previously thought, but we can't definitively draw that conclusion because there's either too much country-by-country variation or we don't have a large enough sample (or both). Finally, coefficients for G and V also become moderate with marginal t-Stats; just like I and M. Therefore our conclusion about the effect of these variables is the same as I and M, but there is probably even more uncertainty with G and V since the addition if just two data points completely changes the result.
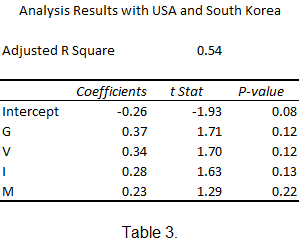
So what does all this mean? It means that the problem is really complicated. What is not complicated is the reality that the homicide rate in the US is 2 to 7 times higher than other peer countries. We are much more likely to kill somebody to solve a problem. This is a fact. But although everybody has an opinion, nobody really knows why. The evidence deflates some arguments, simply says "maybe" on others, and even contradicts itself sometimes. It is easy to see how people on both sides of the guns and violence debate can cherry-pick data to support their position.
My analysis is admittedly overly-simplistic. There are many other independent variables that could be included such as unemployment, drug trafficking and other illegal activity, and gang violence. Gun ownership does not mean the same thing in all countries, as there is large variability in what types of weapons can be purchased, who can purchase them, and when and where they can be used. But simple doesn't make it invalid. Especially considering the whole point of this article was to provide an example of how to minimize confirmation bias, table preexisting beliefs, and use critical thinking skills to base conclusions on facts and evidence.
So what are my conclusions?
1. There simply is not a strong relationship between homicide rates and gun ownership or video games. Violent crime and murder rates have declined for 20 years, while gun rights and gun ownership have expanded, and violent video game playing has come of age. Data analysis across most countries results in effects that are small and statistically insignificant.
2. If there is a relationship between gun ownership and murder rates, it is uniquely American. There is something in the fabric of our country or our cultural psyche that drives us toward confrontation, violence, and guns to solve problems. Although violent crime has declined, our murder rate is still much higher than any other developed country. The mere presence of guns may not be the root cause, but whatever emotions, fears, or anxieties drive us to buy so many guns may be the same ones that drive us to so many killings.
3. Given those facts, I do not support contraction of gun rights on most weapons, as it would have no affect on crime rates. However, I would support increased regulation or bans on certain types of high-capacity assault weapons, with complete understanding that it would have little impact on overall crime. It would make a large percent of the American population feel safer, and that is a valid perspective on our civil rights. I.e. One person's right not to live in fear trumps another's right to say certain things, or to own certain types of weapons. (Interestingly, this same "makes me feel safer" argument is used by many gun advocates to justify concealed carrying of guns.)
4. We need to accept that gun ownership is something fairly unique to our country, just like 3 car garages and Sunday football, and begin to change the dialogue from for or against to safe and appropriate use. The NRA would probably be happy to lead this charge, and this would be the start of shifting the American psyche. Look at how quickly attitudes toward unprotected sex and smoking have changed in just a couple of decades, and I believe a similar shift could occur regarding the use of guns to solve problems.
5. As part of number 4, I would support state-level licensing to own and buy guns, with graduated requirements based on the lethal capacity of the gun. The process and education required to buy and use a large caliber weapon should be at least as rigorous as the one required to get a commercial driver's license. Both help ensure responsible behavior and public safety. This restricts a person's right to bear arms no more than studying and taking a driver's test restricts a person's right to drive.
6. Finally, I agree with Steven Pinker that the discussion on this topic needs to revolve on the 45 people who are murdered each day, every day; not on the rare, Newtown-like massacres. The individual murders are the ones that add up to 16,000 killed per year and the highest murder-rate in the developed world. As hard as it is to acknowledge, mass killings are unpredictable, random events that are nearly impossible to prevent. (However, I might change my mind on this one if 2011 ends up being the start of a trend, as opposed to a typical, random clustering of unlikely events.)
I encourage everybody to take some time, try to put your preexisting beliefs aside, seek objective or well-balanced information, and draw your own conclusions. They may be the same as mine, or different, but in either case they will be informed and provide the foundation for a real, productive discussion. That's what critical thinking is all about.